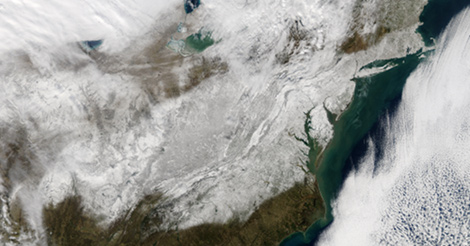
SS asks:
What makes statistical modeling different from, say, new age methods of trying to predict the future?
If you live on the east coast of the United States and paid any attention to the news in the past week, you couldn’t get away from statistical models. There are US models & European models, all trying to answer the same questions: How much snow will fall, in what cities, and when? I live north of Pittsburgh, on the outer edge of this particular storm’s path. One day the models said the storm was tracking north and we should expect half a foot of snow or more; the next day the models said it was tracking south and we might not get any snow. At that rate, why not just flip a coin?
For most of us, weather forecasts are the statistical models we encounter most often. The predictions include some uncertainty, sometimes expressed as a range (2-6 inches of snow) and sometimes as a probability (40% chance or rain). Ranges are straightforward enough, but human intuition is notoriously unhelpful when dealing with probabilities. For one thing, we don’t ever actually experience probabilities; it either rains or it doesn’t. Consequently, it is both hard to grasp what a 40% chance of rain actually means, and to assess from casual observation whether those predictions turn out to be accurate. Factor in the way that forecasts vary from day to day, and from news outlet to news outlet, and the whole enterprise of predicting the weather can seem quixotic. Would reading tea leaves be any better or worse?
Weather models do have the benefit of incorporating causal relationships. For example, we know that when a mass of warm air and a mass of cold air meet, there will be wind. We can measure those air temperatures precisely, frequently, and over wide geographic areas to obtain the necessary input data. Applying that data to models of how air temperature influences wind, and we can predict how air and storm systems will move in the future. In principal, it’s not all that different from how we put men on the moon. We know how the Earth and the moon move, we know how rockets move, and we can measure all the relevant variables. Put all of that together and we can predict where the moon will be and how to get a rocket there at the same time.
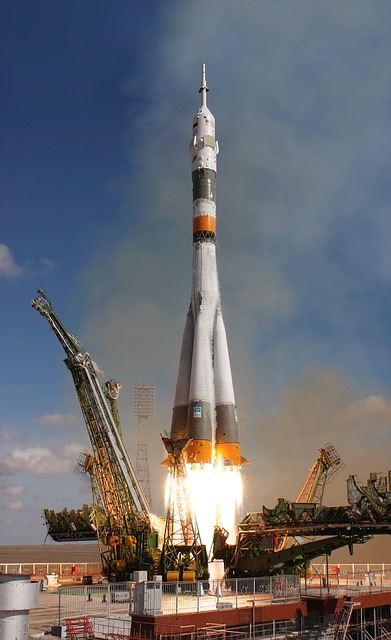
The challenge for weather forecasts is the sheer number “moons” — many trillions of trillions of molecules. We can’t possibly track all of them individually; we have to deal in aggregates–currents and fronts and so forth. This puts us in the territory of statistics. A cold front isn’t a rigidly delineated object, it is the net result of all those molecules responding individually to external factors like gravity and the rotation of the earth. Those factors create correlations and trends in the movements of air molecules that can be used to predict the future behavior of the aggregate front without knowing exactly what each molecule will do. (Just as we can predict overall lottery revenue without know exactly who will win.)
This statistical approach is fruitful over many forecasts, but the exact particulars of a given storm path are sensitive to the specific movements of those individual molecules that we aren’t tracking or modeling. This makes predicting the weather kind of like predicting winning lottery numbers if we knew that the balls were weighted to make certain numbers more likely. We have some information that allows us to do better than blind guessing, but there are still multiple possible outcomes. We can improve the precision of our measurements and build bigger supercomputers to process more detailed data, but some amount of uncertainty will always exist in weather forecasts as long as we can’t watch all the molecules.
These same limitations apply to climate models, which are distinct from weather models but do incorporate some of the same data. We also have more to learn about the causal relationships affecting the climate, since we can observe weather daily and hourly but climate on the scale of years. Nevertheless, we understand the climate well enough that a variety of bets about future (now present) climate conditions are paying off in favor of widely used statistical models.
You may also have encountered statistical predictions about your own health or the health of friends and relatives. We hear about the risks of various behaviors that might increase our chances of getting cancer or diabetes, or about the probability of living so many years after a given diagnosis. The models behind these predictions likewise involve uncertainty. Some of that stems from observational limitations; we can’t peer into your body and see what every one of your cells is doing. Some of the uncertainty is also because our understanding of causal relationships in biology and medicine is more limited than in physics. We can make predictions based on correlations observed in the past, but sometimes those correlations are circumstantial to a certain study and don’t hold for everyone. Steps can be taken when doing research to minimize this possibility, but they aren’t always taken because not everyone is familiar with the statistical nuances.
That doesn’t mean modern medicine or your doctor can’t tell you anything about your future health prospects. It does mean that not all statistical predictions are created equal, and as consumers of those predictions we may have to consider that reality when deciding which ones to put the most stock in. We can also keep in mind that individual medicine will always be a challenge for statistical models; they do well for large numbers of patients but can’t guarantee perfect accuracy for each person. A desire for greater certainty about our own individual situation may lead us to seek answers from more personally attuned sources, but we need to hold those sources to the same accuracy standards as statistical models and be aware that psychological factors may make that personal touch seem more helpful than it really is.
About the author:
Andy has worn many hats in his life. He knows this is a dreadfully clichéd notion, but since it is also literally true he uses it anyway. Among his current metaphorical hats: husband of one wife, father of two teenagers, reader of science fiction and science fact, enthusiast of contemporary symphonic music, and chief science officer. Previous metaphorical hats include: comp bio postdoc, molecular biology grad student, InterVarsity chapter president (that one came with a literal hat), music store clerk, house painter, and mosquito trapper. Among his more unique literal hats: British bobby, captain's hats (of varying levels of authenticity) of several specific vessels, a deerstalker from 221B Baker St, and a railroad engineer's cap. His monthly Science in Review is drawn from his weekly Science Corner posts -- Wednesdays, 8am (Eastern) on the Emerging Scholars Network Blog. His book Faith across the Multiverse is available from Hendrickson.