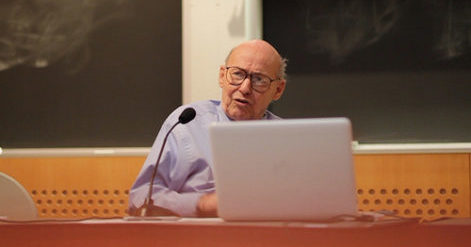

ESN contributor and friend of the blog J. Nathan Matias and some colleagues are writing a series of articles on artificial intelligence to introduce Christian audiences to important topics and themes. They have an introductory essay which will link to additional articles as they appear in the weeks to come. Some of those are already available; for now I wanted to bring the series to your attention, and later this month we’ll discuss the specifics. I’m particularly keen on “Relating to Artificial Persons” as I’m very interested in how our relationships with our simulated human agents interact and influence our relationships with actual humans.
I was particularly struck by this quote from A.I. pioneer Marvin Minsky in 1965: “If one thoroughly understands a machine or a program, he finds no urge to attribute ‘volition’ to it.” I don’t know what Minsky intended 50 years ago, but I can’t help thinking how aptly his comment applies even to human beings. The more we understand our biological and psychological processes, the more we think of them as mechanical or programmatic and the less we attribute ‘volition’ to ourselves. Indeed, it is not hard to imagine a day when computers are deemed to possess comparable intelligence to humans because we have convinced ourselves that our own intellects are entirely computational. Will we have rendered the concept of volition moot in the process? Or will we have discovered a more detailed way of describing what we have always understood as will in the first place? Relatedly, if we learn more about the processes by which God acts, are we lessening our urge to attribute volition to him? Are we eliminating the need to invoke God at all?
On a separate topic, Matias et al introduce the challenge of developing unbiased decision algorithms when the data used to train those algorithms are the result of biased human activities. In many cases, we wind up with algorithms that recapitulate our biases, calling into question whether unbiased results are even possible. Perhaps unbiased decision is something of an oxymoron and we should consider instead what kind of bias we wish to institute.
The discussion of probability, algorithms and bias got me thinking about maximum likelihood methods and the consequences of employing them to develop our machine learning algorithms. Maximum likelihood answers are wrong less often than other approaches by definition, but they can still be wrong. The times when they are wrong will be cases at the margins or in the minority. So what happens when those are people, not just abstract cases? What are the consequences for those people when we choose maximum likelihood approaches? Now, not all machine learning techniques involve maximum likelihood, and algorithms can be evaluated across a range of inputs and outputs. Still, as noted, biases do sometimes wind up in the end result.
I’m not a machine learning expert; these questions are the sincere but perhaps naïve musings of a curious outsider. I look forward to learning more as I follow along with this series and maybe find some answers to my questions or maybe find better questions to ask.
Andy has worn many hats in his life. He knows this is a dreadfully clichéd notion, but since it is also literally true he uses it anyway. Among his current metaphorical hats: husband of one wife, father of two teenagers, reader of science fiction and science fact, enthusiast of contemporary symphonic music, and chief science officer. Previous metaphorical hats include: comp bio postdoc, molecular biology grad student, InterVarsity chapter president (that one came with a literal hat), music store clerk, house painter, and mosquito trapper. Among his more unique literal hats: British bobby, captain’s hats (of varying levels of authenticity) of several specific vessels, a deerstalker from 221B Baker St, and a railroad engineer’s cap. His monthly Science in Review is drawn from his weekly Science Corner posts — Wednesdays, 8am (Eastern) on the Emerging Scholars Network Blog. His book Faith across the Multiverse is available from Hendrickson.
Good article, and worthy effort to consider our technology in relation to our faith. I would suggest reading Mary Shelly’s Frankenstein and watching “Collosus, The Forbin Project”, and Asimov’s Foundation series to give this discussion real depth. Our machines cannot exceed their creators any more than we can develop a perpetual motion machine or cold fusion. They will always reflect us, and in every example to date AI has reflected the weaknesses and prejudice of humanity. Even worse, it starts with most likely outcome as it’s objective, which is the opposite of how human history has developed and how God works in the world. From Tuthmose III to King David, Augustus, Elizabeth I, Napoleon, Hitler, Khomeni, Reagan, Obama and many, many others, the least likely candidate was the person who changed history for better or worse. Technology is not going to change the way the world works, it is going to magnify human weakness and its effects. We have already allowed our technology to exceed our ability to control it. You can’t keep people off their phones in theaters, in moving cars and trucks, in the classroom, in aircraft where it was labled a threat for years, or even at gunpoint at the airport (TSA gave up on this one point of security). If you want to see where AI will take us, watch the original “The Day the Earth Stood Still”. Arming and empowering our technology to kill us all at the planetary level if we use other technologies it to hurt each other at a scale that threatens our neighbors. Same outcome in Collosus, but on a lower level. Both make the point that our aim is not to help man but to create God, this time in our own image…and that is terrifying given that we are evil in intent and retarded in love, and finite in understanding. An omnipotent silicon god created by finite, selfish people…what could possibly go wrong?
Daniel, thanks for these comments. Indeed, science fiction is rife with stories about both the dangers and limits of human creation, and your examples are well-chosen examples. At the same time, as creations such stories also have the potential of being limited by their creators. Does Frankenstein reflect the true limits of what technology can achieve, or does it merely reflect Shelley’s own attitudes and expectations? Her alternate title, The Modern Prometheus, evokes the classical clash between man and god over dominion of the physical realm, yet in a Christian framework God grants man such dominion.
Of even greater interest to me is the question of how her story has subsequently shaped everyone else’s attitudes and expectations. To be sure, a healthy dose of caution, skepticism, and restraint is warranted when it comes to medicine and biotechnology. But has biomedical research been held back simply because the specter of Frankenstein’s monster looms large in our collective imagination to the point where any engineered chimera will likely invoke Shelley’s tale?
I think it is right to say technology magnifies and amplifies our capabilities, both for good and ill. But in doing so, it does change how the world works if for no other reason than because we can. We, and indeed all living creatures, have the ability to change our environment and technology has allowed us to do so on such a grand scale that we have changed the global climate. Our communication tools are shaping our minds and perhaps even rewiring our brains.
Technology also has the ability to obfuscate causality by amplifying complexity. As you note, there are already unintended consequences on various scales resulting from our inability to anticipate the consequences of our innovations and their applications. And via deep learning (and possibly other techniques), we have already developed AI that we cannot fully explain the workings and behavior of. That doesn’t mean we can extrapolate to some sort of omniscient or omnipotent AI, but it suggests that perhaps we can create entities that exceed us in some fashion.